Artificial Intelligence
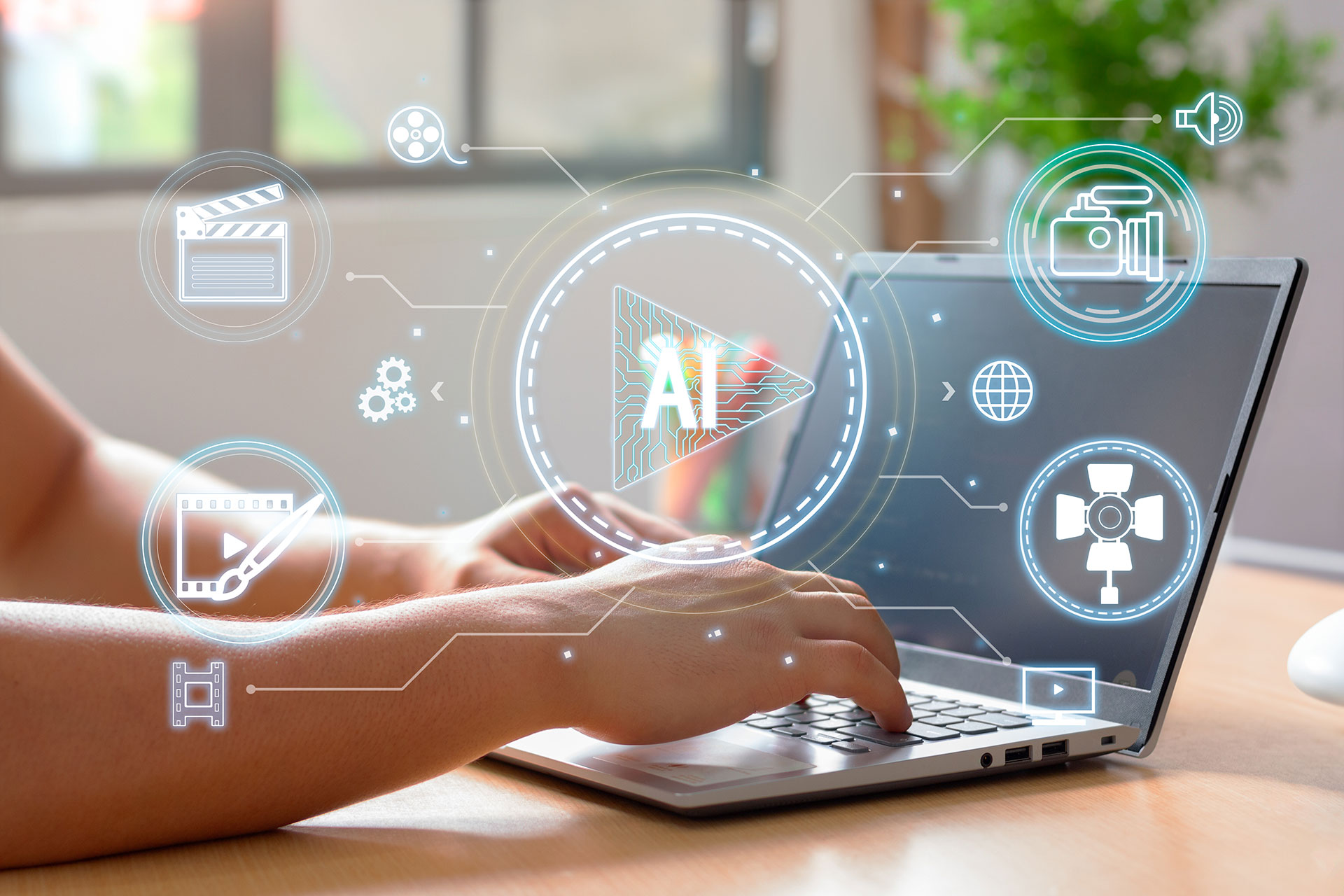
The period before 1950
The 'McCulloch and Pitts Neuron’ is a mathematical model proposed in 1943 to represent conduction in nerve cells in order to understand the human mind and decision-making process. This algorithm architecture forms the basis of popular artificial neural networks today.
British mathematician Alan Turing suggested asking the question 'Can machines think?' in his article published in 1950. ‘The Imitation Game' described in the article is about a human controller asking questions through text-based communication with a human and a machine in different rooms, and the machine is trying to convince the controller that it is human. Thus, it was stated that if a machine can compete with a human on equal terms (text-based communication) and deceive the human controller, it can be said that there is intelligence. In this game, for which different alternatives have been developed over time, the developers have deliberately produced systems that make human errors (e.g. late typing, wrong answers), which has called into question the perception that 'machines are never wrong'.
The period between 1950-1970
The term 'Artificial Intelligence' was first used in a workshop organized by John McCarthy in 1956 in Dartmouth. This name aroused a higher level of curiosity in society than researchers expected, but it also raised expectations from related technologies. It is worth thinking about whether the interest and development in these technologies would have been the same today if a less interesting name such as 'computable learning' had been chosen instead of 'artificial intelligence' back then.
In 1958, Ord. Prof. Dr. Cahit Arf gave a speech on 'Can machines think and how can they think?' at the event of public education conferences held at the opening of the academic year of Atatürk University. In the same year, the previously proposed 'McCulloch and Pitts Neuron' led to the development of the 'Perceptron' algorithm, and in 1962, the design of the multi-layer perceptron architecture was suggested. Although this model still lacked deep learning elements, it was defined as 'extreme machine learning' for its time. In 1965, Stanford University had a project called DENDRAL and the Massachusetts Institute of Technology had a project called ELIZA.
The period between 1970-1990
In 1974, Stanford University launched the SUMEX-AIM project, which shared computer resources by using national network infrastructures such as ARPANET. For example, the MYCIN project is an expert system developed to make recommendations for choosing appropriate antibiotics for the patient. This rule-based system, developed with the opinions of experts who were not sufficient in number at the time, was designed as a tool to help less experienced physicians choose the right antibiotic. Later, new software based on the MYCIN project was developed, and instead of antibiotic elements, a common platform was produced where developers could design expert systems in any scenario they wanted. In his master's thesis in 1986, Fikret Uluğ transferred the EMYCIN software to a different programming language to build a similar platform and developed two different expert systems for ‘car engine problem diagnosis' and 'financial advices'.
The period after 1990
These systems were first created in theory and then developed with the technological possibilities of that day. What the developed systems could do was limited and the margin of error was higher than today's. Therefore, special care was taken to ensure that the decisions taken by the machine were explainable. In some periods, developments came to a halt and there were periods of stagnation called 'artificial intelligence winter'. Over time, the computing power of computers increased, and by the 90s, machine learning techniques such as deep learning were developed. In the 2000s, convolutional neural network models were introduced and gained attention with their success in image processing. The process, which started with the identification of objects such as cats and dogs, continued with serious advances in different subjects such as autonomous driving. In 2016, the AI system called AlphaGo, developed by DeepMind, declared its victory to the whole world by beating the world champion in a five-game competition in the game called Go. However, this software was developed specifically for this game, and its superiority over humans is also within the scope of this game. However, it is not possible to play another game such as chess or sudoku, or make clinical decisions with the same software unless it is included in the training.
Development of AI
Historically, studies aimed at unraveling the human mind led researchers to the question of how to transfer a mind into a machine. Then, algorithms were developed to calculate the desired output by analyzing the problem mathematically. Not to forget, the perception of these systems about the universe we live in is limited only by the data presented to it. Moreover, these data are often just numbers and have no idea what they actually are. This situation requires careful use of the relevant systems. Big data can be evaluated tirelessly with AI, and many improvements can be achieved in the field of health. Thus, perhaps better-quality images can be obtained by improving the data coming from an imaging device's sensor, but with today's technology, the final decision is still up to the user. In addition, although today models such as deep learning or convolutional neural networks achieve high success in some tasks, they are defined as 'closed boxes' because the mechanism of the decisions they make based on statistical calculations cannot be fully explained. However, the development of new architectures such as generative adversarial network (GAN) is exciting.
Position of AI in healthcare
In summary, AI is a tool and can be useful when used correctly. It does not get tired like a human, it does not make decisions based on its emotions, but both can 'make mistakes'. Today, we use different AI systems in daily life, whether consciously or unconsciously. The digital transformation in healthcare and the increase in digital patient data produced facilitate the development of various AI applications, as integrated into the system or independent software. The usage area of these technologies is not limited to the clinical decision support systems. For example, similar systems can be used in the stock management of a healthcare facility or in the organization of healthcare services across the country. Artificial limbs can be controlled by processing electrical signals collected from the brain. Although it is still a distant dream to leave the entire process to robots with AI in the field of healthcare, today, knowing the advantages and disadvantages of these systems plays a key role in increasing the benefits obtained from relevant technologies.
Misconceptions
AI does not make mistakes.
Artificial intelligence applications are simply a statistical analysis of numerical data, and the results may contain errors. Although its ability to quickly learn large volumes of data makes it look smart, that doesn't mean that it won't make any mistakes.
An intelligent AI system can be dangerous if it learns new things by hiding them from humans.
Today, artificial intelligence models are trained for a purpose and it is not possible to transfer their knowledge from one subject to another subject (unless programmed for that). Moreover, most software is delivered after the training has been completed by the developers and the model is no longer learning anything new. Although some models update themselves with data from the user (if included in features), these are limited to purposeful topics. Unless a model is developed to cause harm, especially by learning new things, these systems are safe, at least in intent.
The complex structure of artificial intelligence makes it difficult to understand its emotions and can be dangerous if hurt.
The production of models that can understand and imitate human emotions is an interesting but difficult topic. Unless this is involved in its training, its sole purpose is to perform the desired task and is independent of emotional decisions.
Artificial intelligence may take our jobs in the future.
It is not possible to predict the future with certainty, but it would be useful to talk about different possible scenarios. These technologies will change professions rather than ending them and may put those who are late in adapting to professional life at a disadvantage. Perhaps, it can support the work to be completed faster and reduce working hours or the number of personnel of companies can be reduced. However, it is possible that new professions will emerge in the coming years. Although the acceleration in technological developments is dizzying, trying to understand these developments rather than closing ourselves off is probably the best thing to do.
1. Orhan, K., Amasya, H. Tıptan Diş Hekimliğine Yapay Zeka. İçinde: Orhan, K. Jagtap, R. (Eds) Diş Hekimliğinde Yapay Zeka. Springer, Cham. (2023).
2. Mcculloch, W.S., Pitts, W. A Logical Calculus Of The İdeas İmmanent İn Nervous Activity. Bulletin Of Mathematical Biophysics 5, 115–133 (1943).
3. AM. Turıng, I.—Computıng Machınery And Intellıgence, Mind, Volume Lıx, Issue 236, October 1950, 433–460, (1950).
4. Mccarthy J, Et Al., A Proposal For The Dartmouth Summer research project on artificial intelligence, august 31, 1955. (2006).
5. Moor J, The Dartmouth College artificial intelligence conference: The next fifty years. (2006).
6. Arf, C. Makine düşünebilir mi ve nasıl düşünebilir. Atatürk Üniversitesi-Üniversite Çalışmalarını Muhite Yayma ve Halk Eğitimi Yayınları Konferanslar Serisi, (1), 91-103. (1959).
7. Rosenblatt F, The perceptron: a probabilistic model for information storage and organization in the brain. (1958).
8. Rosenblatt F, Principles of Neurodynamics Spartan. (1962).
9. Weizenbaum J, ELIZA—a computer program for the study of natural language communication between man and machine. (1966).
10. Lederberg J, Systematics of organic molecules, graph topology and Hamilton circuits. A general outline of the Dendral system Interim report. 1966.
11. Freiherr G, The seeds of artificial intelligence: SUMEX-AIM: US Department of Health, Education, and Welfare, Public Health Service, (1980).
12. Van Melle W, MYCIN: a knowledge-based consultation program for infectious disease diagnosis. (1978)
13. Weiss S, The EXPERT and CASNET consultation systems. (1979).
14. Ulug F, Emycin-Prolog expert system shell. 1986, NAVAL POSTGRADUATE SCHOOL MONTEREY CA.
15. LeCun Y, et al., Gradient-based learning applied to document recognition. (1998).
16. Wang F-Y, et al., Where does AlphaGo go: From church-turing thesis to AlphaGo thesis and beyond. (2016).
17. Yüce, F, Taşsöker, M. Diş hekimliğinde yapay zeka uygulamaları. 7tepe Klinik Dergisi, 19(2), 141-149. (2023)
18. Zaim Gökbay, İ. Tıpta yapay zeka uygulamaları-antik çağdan yapay zekaya teşhis ve tedavi yöntemlerinin gelişim sürecinde klinik karar destek sistemlerinin evrimine genel bakış. İstanbul Üniversitesi Yayınevi, İstanbul, 673-692. (2021)